Working of Image Recognition Technology
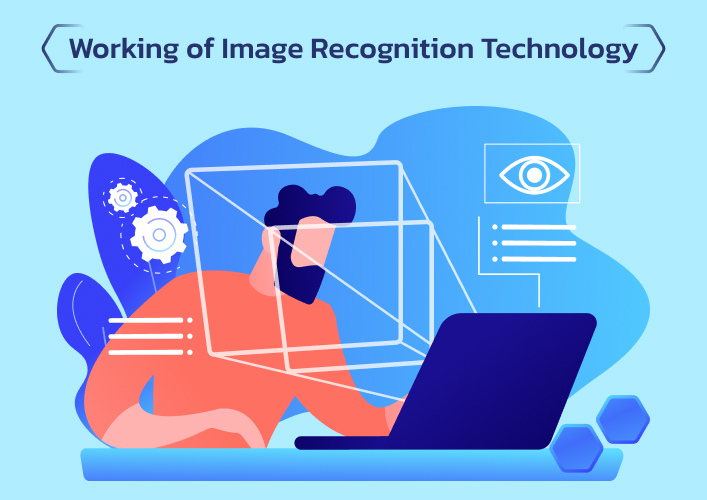
In the context of digital photographs, ‘image recognition’ refers to the technology that can pinpoint certain locations, people, objects, and structures. Humans have a remarkable capacity to recognize many visuals, including those of animals. We can readily distinguish the image of a cat and separate it from an image of a horse. But a computer could find it difficult to do so.
Pixels, the building blocks of digital images, are square or rectangular picture parts that have a specific numerical value for their intensity or grayscale level. A computer interprets a picture as a collection of numerical values for individual pixels, and in order to identify a specific image, it must first identify the recurring structures within those numbers. Let’s understand how it works.
Image recognition is often accomplished by training a model to analyze the image’s pixels. As many labeled photos as possible are put into these models in order to teach them to identify other images. A dataset of photographs and their labels is required. For example, an image of a dog must be tagged as a dog.
After that, a model will be trained using these photos as input. Convolutional neural networks are commonly used for image-related applications. In addition to Multiperceptron (MLP) layers, these networks also have “convolutional” and “pooling” layers. During training, the visual recognition model learns to identify features and patterns in the images from the dataset. After feature extraction, fully connected layers map these features to specific classes or categories.
Learning Types of Image Recognition Technology
Supervised learning, unsupervised learning, and self-supervised learning are the three methods that may be used to train an image recognition system. Typically, the key difference between the three training methods is how the training data is labeled.
- To classify images into their respective categories, supervised learning algorithms are utilized in this method of image recognition. By assigning labels like ‘car’ and ‘not car’, a human may teach a visual recognition system to identify vehicles. In supervised learning, the data is categorized before being fed into the system.
- In unsupervised learning, a visual recognition model is presented with a dataset of photos without any labels, and it must figure out the key similarities and differences between the images by analyzing their properties and characteristics.
- Similar to unsupervised learning, self-supervised learning makes use of unlabeled data. Pseudo-labels derived from the training data are used to facilitate the learning process in this model. This method may be helpful in situations when labeled data is sparse since it enables computers to learn to represent the data with less accurate data. For example, self-supervised learning may be used to educate a computer to replicate human faces. When given fresh information after the algorithm has been trained, it produces whole new faces.
If you have access to labeled data and already have an idea of the kind of recognition you need to do, supervised learning may be a helpful technique. Unsupervised learning is beneficial when the categories are unknown and the system has to find similarities and differences between the pictures. When there is a lack of labeled data and the machine must learn to represent the data with less accurate data, self-supervised learning is a beneficial technique.
Other Common Types of Visual Recognition Techniques
Other prominent examples of image recognition methods include:
- The most popular kind of visual recognition is called ‘object recognition’ and it entails locating and labeling things in a picture. Object recognition has several potential uses, including the detection of manufacturing faults in items and the identification of species in wildlife photographs.
- Recognizing people by their faces is called ‘facial recognition’ a subset of object recognition used to confirm their identities. Multiple fields, including security, surveillance, advertising, and law enforcement, may benefit from facial recognition technology.
- The process of scene recognition entails determining what kind of scenario is shown in a photograph and then classifying it accordingly. Autonomous cars, augmented reality, and robots are just a few of the many uses for scene recognition technology.
- OCR, or optical character recognition, is a subset of image recognition that focuses on extracting and converting text from pictures for use by computers. Text from scanned documents may be extracted using OCR and then converted into searchable digital text for use in document management systems.
- In order to engage with technology or gadgets, gesture recognition identifies and interprets human gestures like hand motions or facial expressions. Games, robots, and augmented and virtual reality are just some of the areas where gesture recognition has found usage.
Conclusion
In conclusion, image recognition technology has developed with several uses in many fields. Automation, efficiency, and creativity may all take a leap forward if robots can read and comprehend visual data. However, it is critical to think about ethical and privacy considerations connected to the use of this technology. It is essential for the ethical deployment of these technologies to strike a balance between technical progress and ethical issues.
FAQs
What is image recognition?
How does image recognition work?
What are some common use cases of image recognition?
- Healthcare: Diagnosis of medical conditions through medical imaging.
- Retail: Product recognition for inventory management and customer experience.
- Security: Facial recognition for access control and surveillance.
- Automotive: Object detection for autonomous vehicles and driver assistance systems.
- Social Media: Content moderation and image tagging.
What are the challenges associated with image recognition?
- Data Quality: The quality of training data directly impacts the performance of visual recognition models.
- Ethical Concerns: Privacy issues and biases in visual recognition systems.
- Computational Resources: Training and running complex models may require significant computational power.
How accurate are image recognition systems?
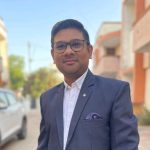
Ravi Bhojani is the Chief Marketing Officer (CMO) at Alian Software, where he spearheads the company’s marketing strategies and drives its brand presence in the competitive IT services landscape. With over a decade of experience in the technology and marketing sectors, Ravi has consistently demonstrated his ability to blend innovative marketing techniques with deep industry knowledge to deliver outstanding results.